Retail is detail, on a massive scale. Retailers need to keep billions of forecasts up to date, control tens of millions of inventory flows and manage tens of thousands of people working in stores and distribution.
Retail is also very dynamic. Each day, retailers need to deal with exceptions ranging from late shipments to quality issues. Moreover, the ongoing transformation of retail impacts all parts of the business. Retailers are developing new ways of serving customers and fulfilling orders, experimenting with new store formats and making investments in technology and robotics. Finally, megatrends, such as urbanization and consumers’ growing interest in healthier and more sustainable products, are changing shopping patterns.
To meet these challenges, retailers have to make a massive number of quick decisions. They know they need to make their data work for them to get all these decisions right. Yet, many are struggling. Although most retailers are rich in data, many are poor in analytics. Access to data does not automatically enable retailers to make better decisions faster. This is where decision science steps in.
Retail Winners Turn Data into Better and Quicker Decisions
Retailers need to grow much better at turning vast amounts of data into accurate, quick and automated decisions. This need is fueling the current hype around AI (artificial intelligence) in retail. Unfortunately, no magic black box is available to solve all of retail’s complex, real-life planning problems.
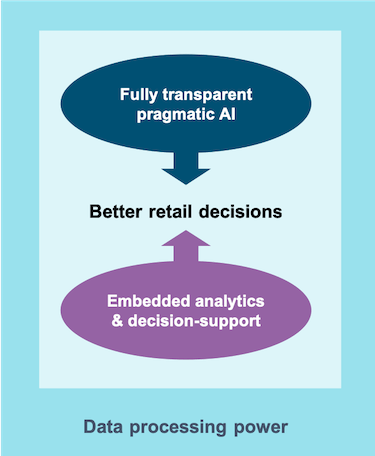
For best results, retailers need to use the full range of pragmatic AI. They need to combine artificial intelligence and machine learning with statistical modeling and optimization, simulation, classification, as well as rules and heuristics.
The right AI approach depends on the type of problem (such as prediction vs. optimization) as well as the available data. Other important factors include computational efficiency and available data processing power. Slow data processing may result in outdated recommendations. In the worst case, decisions need to be made before the calculations are ready, putting AI out of play.
Data and math are powerful tools, but computers are not yet capable of running complex planning processes entirely on their own. This is especially true in the turbulent world of retail. There is still an important role for human intelligence.
Expertise is needed to define business priorities and resolve ill-defined or unprecedented problems. Intelligence amplification (IA) — the part of decision science leveraging human expertise — is as important as AI.
Pragmatic AI for Accuracy and Automation
Retail planning encompasses a wide range of situations and decisions. For example, long-term forecasting, smoothing of inventory flows and optimized ordering of fresh products are very different types of problems. No single method or algorithm applies to all of these situations.
The full range of pragmatic AI is needed to make sense of retail data and turn it into recommendations and autonomous decisions. This includes artificial intelligence and machine learning algorithms, but also advanced statistical modeling, optimization, simulation and classification as well as rules and heuristics. (See Figure 2 for real-life examples of all these approaches.)
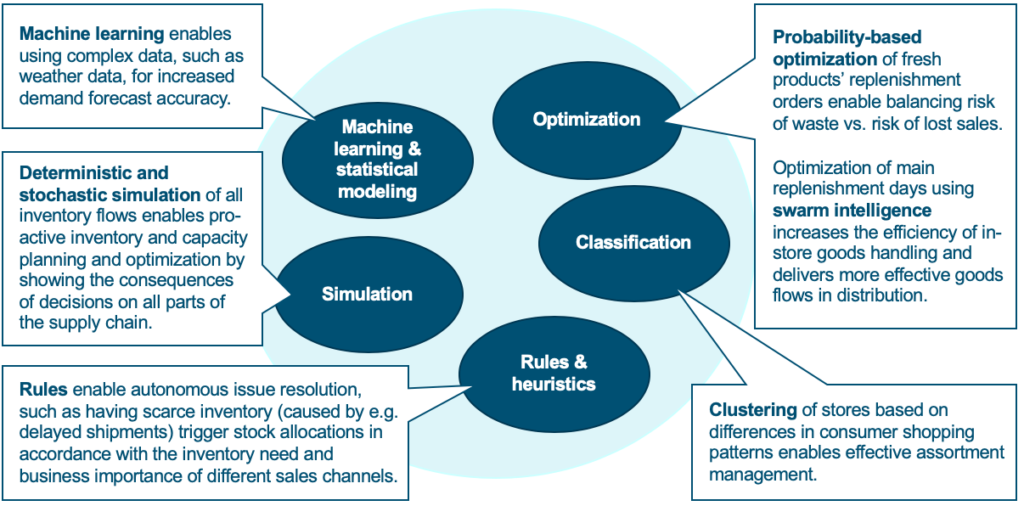
When applied correctly, the results of pragmatic AI are astounding: improved profit margins, increased sales, reduced waste and more effective inventory and capacity utilization. The benefits to the retailer, its customers and the planet are immense. (Read about the results attained by German grocery retailer Bünting for inspiration).
The use of pragmatic AI, however, needs to be carefully designed:
- The right methods and algorithms need to be selected automatically based on available data and type of problem
- All AI approaches must be reviewed and revised as technology develops and new data become available
- The methods and algorithms need to operate efficiently and reliably at retail-scale, enabling effective management of retail’s billions of forecasts and tens of millions of goods flows
- All pragmatic AI approaches need to work seamlessly together and support cross-functional planning
- Its reasoning must be completely transparent, allowing users to fully understand, trust and sometimes adjust calculations and results, without being data scientists
Decision Support and Analytics for Leveraging Human Expertise
Access to more data, better algorithms and increasing data processing power are making decision-making more granular, more accurate and more automated. Still, there is demand for planning expertise.
New situations, with limited data, arise as retailers are reinventing themselves. Human expertise is important when retailers plan new store formats or new services. Similarly, many complex issues are best resolved by human planners discussing available options with category managers or suppliers. Finally, planning expertise is essential for translating each retailer’s unique business strategy into the detailed objectives that control how AI optimizes outcomes.
Support for turning data into information and recommendations — intelligence amplification — is needed to reap the full benefits of planner expertise. It also makes the planners’ work more meaningful as it allows them to spend less time on routine data preparation and more time on actually making decisions. Planning tools, thus, must have powerful analytics built into them. In this way, no time is wasted switching between systems or waiting for reports when decisions need to be made.
Intelligence amplification in retail planning means that planners can:
- Trust the system to alert them of issues that the system cannot resolve on its own
- Access all data in the same system where the planning work takes place
- Analyze data by drilling down into details or by looking for more general patterns as needed, with complete flexibility, in real-time and with the aid of powerful visualizations
- Run and compare what-if scenarios on the fly to test the impact of complex decisions on each part of the supply chain and across functions
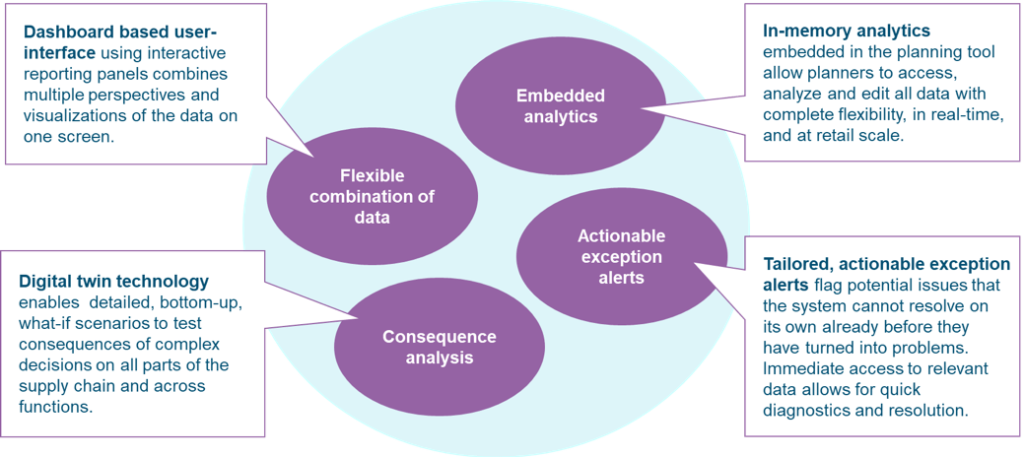
Retail Winners Go Beyond AI
No business transformation will follow from adding a few isolated AI applications to a retailer’s planning processes. Quicker and better decisions require that retailers take advantage of the full breadth of decision science. Data science, which focuses on making sense of data by using statistical analysis and algorithms, is not enough. To enable better decisions, the interdisciplinary application of business, math, technology, design thinking and behavioral sciences —decisions science — is needed.
In closing, consider this compelling argument from Dhiraj Rajaram: “Decision science enables addressing business problems that are ill defined and shifting and where the factors affecting the problem are not completely understood. It facilitates the design thinking paradigm: Taking business problems that start off as a hunch or as mysteries to becoming heuristic, rules and judgment based, to becoming algorithm as one starts to see patterns, to becoming codified and tool-ified in parts before being operationalized in systems. Further it enables the on-going creation, translation and consumption of data-driven insights to help organizations make better decisions. Decision science integrates and builds on data sciences by adding the aspects of business context, design thinking and behavioral sciences.” Exactly what the doctor ordered, isn’t it?
List of resources
Pushing the boundaries of data processing requires specialization explains the technology behind high-performance analytics and discussed its use in retail planning.
Winning the Food Fight: Best Practices for Managing Grocery Retail Supply Chains presents several real-life examples of pragmatic AI.
Why some data scientists should really be called decision scientists by Dhiraj Rajaram discusses the difference between data science and decision science.